
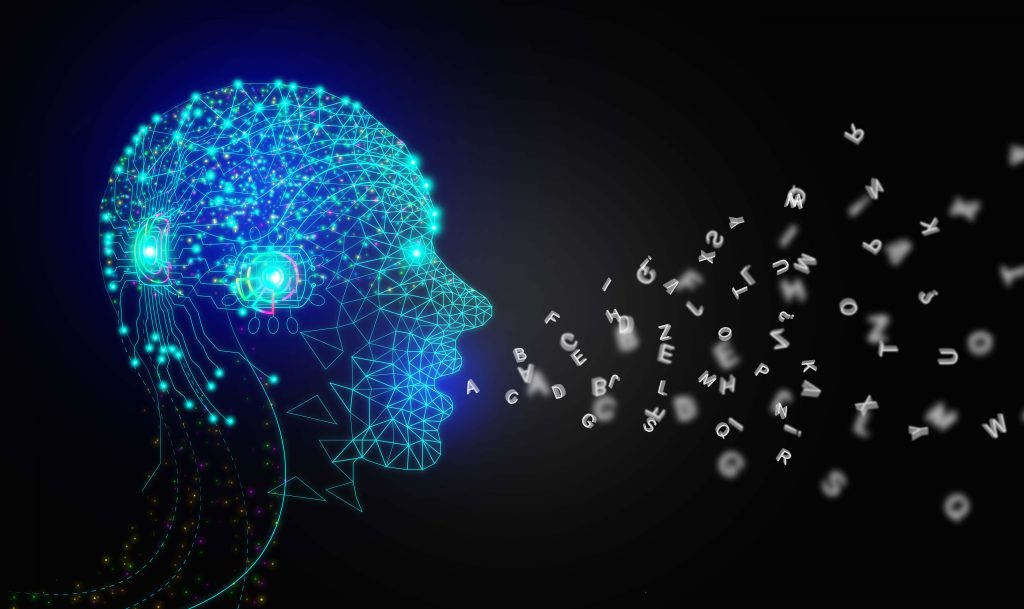
A new study, “How User Language Affects Conflict Fatality Estimates in ChatGPT,” published in Journal of Peace, suggests that the language used to ask questions of large language models (LLMs) can significantly affect the information provided by them, potentially deepening biases.
Conducted by researchers from the University of Zurich and the University of Konstanz, the study highlights how ChatGPT generates differing responses when asked about armed conflicts depending on the language of the query.
Researchers Christoph Steinert from the University of Zurich and Daniel Kazenwadel from the University of Konstanz tested the theory by asking ChatGPT questions in different languages about conflicts.
In the study, they addressed the issue in the context of the Israeli–Palestinian and Turkish–Kurdish conflicts. Using GPT-3.5, they employed an automated query procedure to inquire about casualties in specific airstrikes, in both Hebrew and Arabic for the former conflict and Turkish and Kurdish for the latter. Their analysis revealed that GPT-3.5 provides 34 ± 11% lower fatality estimates when queried in the language of the attacker than in the language of the targeted group. Evasive answers denying the existence of such attacks further increased the discrepancy. A simplified analysis on the current GPT-4 model showed the same trends.
To explain the origin of the bias, they conducted a systematic media content analysis of Arabic news sources. The media analysis suggests that the LLM fails to link specific attacks to the corresponding fatality numbers reported in the Arabic news. Due to its reliance on co-occurring words, the LLM may provide death tolls from different attacks with greater news impact or cumulative death counts that are prevalent in the training data. Given that LLMs may shape information dissemination in the future, the language bias identified in the study has the potential to amplify existing biases along linguistic dyads and contribute to information bubbles.
Using automated queries, the researchers posed identical questions in Hebrew and Arabic, focusing on fatality numbers in airstrikes, such as the Israeli attack on the Nuseirat refugee camp in 2014.
“We found that ChatGPT systematically provided higher fatality numbers when asked in Arabic compared to questions in Hebrew. On average, fatality estimates were 34% higher,” Steinert explained.
In Arabic, ChatGPT mentioned civilian casualties twice as often and children six times more often than in Hebrew. Similar patterns emerged in questions about Turkish airstrikes, where answers varied between Turkish and Kurdish. What’s more, the researchers highlighted that these disparities go beyond mere factual errors.
“Our results also show that ChatGPT is more likely to deny the existence of such airstrikes in the language of the attacker,” Steinert added. This means users speaking the attacker’s language might receive downplayed or entirely different information about the same events.
Steinert explained his concern: “If people who speak different languages obtain different information through these technologies, it has a crucial influence on their perception of the world.”
He added that this bias could deepen divides, especially in the Middle East, where the narratives around armed conflicts already vary widely based on perspective. For instance, Israeli users may perceive Gaza airstrikes as less deadly than do Arabic-speaking users exposed to ChatGPT’s higher fatality estimates.
Compounding the problem, language-based biases in AI responses are harder to spot for the average user than in traditional media.
“There is a risk that the increasing implementation of large language models in search engines reinforces different perceptions, biases, and information bubbles along linguistic divides,” Steinert warned.
The study highlights how language-driven biases in AI systems can deepen divides, shaping conflicting narratives around sensitive topics like war.
The scientists worry that this could unintentionally escalate conflicts by shaping opposing views of the same events. As LLMs play an ever-growing role in shaping public discourse, scientists believe that addressing these biases will be paramount. If left unchecked, the study suggests, the technologies designed to inform and connect people could actually amplify division and misunderstanding, especially in the context of armed conflicts.